2009
Volume 3 Number 1
Classics in the Million Book Library
Abstract
In October 2008, Google announced a settlement that will provide access to seven million scanned books while the number of books freely available under an open license from the Internet Archive exceeded one million. The collections and services that classicists have created over the past generation place them in a strategic position to exploit the potential of these collections. This paper concludes with research topics relevant to all humanists on converting page images to text, one language to another, and raw text into machine actionable data.
Introduction
In a long span of time it is possible to see many things that you do not want to, and to suffer them, too. I set the limit of a man's life at seventy years; these seventy years have twenty-five thousand, two hundred days, leaving out the intercalary month. But if you make every other year longer by one month, so that the seasons agree opportunely, then there are thirty-five intercalary months during the seventy years, and from these months there are one thousand fifty days. Out of all these days in the seventy years, all twenty-six thousand, two hundred and fifty of them, not one brings anything at all like another. (Herodotus, Histories 1.32, tr. Godley)
From Curated Collections to Dynamic Corpora
- Massive scanning. In December 2004, Google seized the initiative to create a vast library of scanned books, with text generated by OCR, but the library community has the resources to convert its print holdings into digital form: the 123 North American libraries who belong to the Association of Research Libraries spent more than a billion dollars on their collections in the 2005-06 academic year.[8] Of course, most libraries will claim that they are under-funded and cannot maintain their existing collections, much less consider a major new initiative. Some of us are old enough to remember hearing that the costs of print collections would never allow for libraries to make digital materials accessible.
- Scanning on demand. The OCA has created an infrastructure whereby individuals could, by 2007, select particular books for scanning and then inclusion in the larger OCA collection. The quality is high and the cost is low: $.10US per page plus handling costs of $5US per book — about $40 for a standard book with about 300 pages. It costs about the same to create a high resolution scan that anyone attached to the internet can scan than it does to buy a single printed book ($52 in 2005-06). Support from the Mellon Foundation has allowed the Cybereditions Project at Tufts University to begin creating within the OCA an open source library of Greek and Latin that will contain at least one text or fragment of every major surviving Greek and Latin author and a range of reference materials, commentaries and core publications.
- The growth of open access and open source licensing. In 2008, open access publication became the dominant model for academic publication. The US National Institutes of Health (NIH) have established a public access policy. As of April 2008, this policy “requires scientists to submit final peer-reviewed journal manuscripts that arise from NIH funds to the digital archive PubMed Central upon acceptance for publication.” [9] The policy further requires scientists funded by the NIH to include in their papers citations to the open access copies of previous publications in the open access PubMed Central web site. The NIH provides more than 22 billion dollars in funding for medical research.[10] Publishers in the most heavily funded research area in all of academia must now develop business models that assume open access that precedes publication. The US NEH, by contrast, requested just under $145 million dollars in funding for 2009 — less than 1% as much as the NIH invests.
- Improved OCR. Traditionally, classical Greek has been a huge barrier for classicists — there was no useful OCR. All classical Greek required manual data entry and such specialized work usually cost much more than data entry for English. By early 2008, Google had begun to generate initial OCR text from page images of classical Greek. The software is evidently based on OCR designed for modern Greek and contains errors, but clever search software could ameliorate this problem. If classicists have access to the scanned page images and can optimize OCR software for classical Greek, we can achieve character level accuracy (99.94%) comparable to the standard quality for manual data entry (99.95%). In a preliminary analysis of printed scholarly editions, we found that 13% of the unique Greek words on a page, on the average, only appeared in the textual notes. Restricting our analysis to older volumes from the Loeb Classical Library (which traditionally provided a minimal number of textual variants), we found that only 97% of the unique Greek words on a given page appeared in the main text. Thus, collections that contain perfect transcriptions of the reconstructed text but no textual notes offer at most 97% — and, if we use fuller editions, 86% — of the relevant data. The worst OCR error that we measured (98%) matches the overall recall rate of perfect transcriptions of text alone.[11] Once we enter multiple editions of the same text, we can begin using each scanned edition to identify OCR errors and intentional textual variants.
- A new generation of text mining and quantitative analysis. The DCB contains 600,000 bibliographic entries from 1949 to 2005 and adds 12,500 new items each year.[12] By contrast, the CiteSeer system, upon which computer scientists depend, was developed more than a decade ago in 1997 at the NEC Research Institute in Princeton, NJ, and offers an automatically generated index of 767,000 publications, including automatically extracted bibliographic citations.[13] Research continues and new generations of automated bibliographic systems, based on the automated analysis of on-line publications, have begun to appear. The Rexa System, developed at the University of Massachusetts, had assembled a collection of almost 1,000,000 publications in 2005 [Mimno 2007]. David Mimno, one of the authors of this paper, is a member of that research group and has support from the Cybereditions project at Tufts University to begin in 2008-09 applying that research to publications from classics.
- Multitexts: Scholars have grown accustomed to finding whatever single edition a particular collection has chosen to collect. In large digital collections, we can begin to collate and analyze generations of scholarly editions, generating dynamically produced diagrams to illustrate the relationships between editions over time. We can begin to see immediately how and where each edition varies from every other published edition.
- Chronologically deeper corpora: We can locate Greek and Latin passages that appear anywhere in the library, not just in those publications classicists are accustomed to reading. We can identify and analyze quotations of earlier authors as these appear embedded in texts of various genres.
- New forms of textual bibliographic research: We can automatically identify key words and phrases within scholarship, cluster and classify existing publications, generate indices of particular people (e.g., Antonius the triumvir vs. one of the many other figures of that name, Salamis on Cyprus vs. the Salamis near Athens). Such searches can go beyond the traditional disciplinary boundaries, allowing students of Thucydides, for example, to analyze publications from international relations and political philosophy as well as classics.
- How representative is the corpus? Is all of a given corpus available on-line? (e.g., have all the published volumes of a series been scanned?) Can we estimate the percentage of the corpus that survives? (e.g., what percentage of Sophocles’ do the seven plays and other fragments constitute?) What biases are inherent in our data? (e.g., do we have any accounts produced by women or by members of every national/ethnic group involved in a topic? If we find 100,000 instances of the Latin word oratio, what are the periods, genres, locations, and (in the case of later Latin) original languages of the authors?)[14] And, are there correlations between these parameters? These may in fact be automatically discernable from the data, even if the human eye doesn’t notice them in the forest of data.
- How accurate are the digital surrogates for each object? We may have a satisfactory corpus of print materials but these materials may yield very different results to automated services such as OCR, named entity identification, cross-language information retrieval, etc. Readers of Jeff Rydberg-Cox’s contribution to this collection will realize that OCR software will, at least in the immediate future, extract much less usable text from early modern printed editions than from editions printed in the early twentieth century. We need automatically generated metrics for the precision and accuracy of each automated process on which we depend.
Services for the humanities in very large collections
- Access to images of the physical sources: This includes access to particular copies of a document, any pagination or naming scheme with which to address the individual pages, and a coordinate system with which to describe regions of interest on a given page. Many born-digital publications do not provide such access — logical “page 12” of a report (as printed as a page number) may physically be page 21 of the PDF document (after adjusting for front matter, a table of contents etc.). Coordinate systems must have sufficient abstraction so that they can address relationships of the printed page even if the paper has been cropped or varies from one printing to another: coordinates for one First Folio should be useful with others.[15]
- Access to transcriptional data: At the least, we need to be able to analyze the words and symbols that are encoded on the physical page.[16] The rough “bag-of-words” approach, where systems ignore the location of words on the page and even their word order, has proven remarkably useful. This level of service is fundamental to everything that follows. Conventional OCR software has traditionally provided no useful data from historical writing systems such as classical Greek. Latin is much more tractable but OCR software expecting English will introduce errors (e.g., converting t-u-m, Latin “then,” into English t-u-r-n). Even earlier books with clear print will contain features that confuse contemporary OCR (e.g., the long ‘s’ which looks like an ‘f,’ such that words such as l-e-s-t become l-e-f-t).[17]
- Access to basic areas of a page such as header, main text, notes, marginalia: Even transcription depends upon basic page layout if it is to achieve high accuracy: we cannot transcribe individual words unless we can automatically resolve hyphenization and this in turn implies that we can distinguish multi- from single column text, footnotes, headers marginalia, etc. from main text, etc.[18] We need, however, to recognize basic scholarly document layouts: thus, we should be able to search for either the reconstructed notes or the textual notes at the bottom of the page. This stage corresponds roughly to WYSIWYG markup. At this stage, the system can distinguish the main text from the notes in Figure 5 and Figure 10 but it does not recognize that one set of notes are commentary and the other constitute an apparatus criticus.
- Access to visually labeled structures within the text: Explicit labeling in this case includes headwords of dictionaries and encyclopedias and canonical citations such as book/chapter/verse/line. These structures draw upon typographical conventions: e.g., bold and indenting to show headwords, numbers in the margins or embedded in the text with brackets to illustrate citations. This stage would recognize where index entries begin along with their headwords and easily recognized citations. This stage corresponds to semantically meaningful structural markup, e.g., descriptive structures about the text.[19] At this stage, the system recognizes that the notes in Figure 5 are a commentary and contain comments on agro vectigali, cum et maxima … ageretur, and tibique … indicium within the text above.
- Access to knowledge dynamically generated from analysis of explicitly labeled knowledge: This process can begin with very coarse analysis: if we recognize when various encyclopedia articles describing several dozen figures named Antonius or Alexander begin and end, then we can analyze the vocabulary of each article to begin deciding which Alexander is meant in running text. This stage includes the lemmatization and morphological analysis to support the lookups and searches familiar to classicists for more than a decade (e.g., query fecisset and learn that it is the pluperfect subjunctive of facio, “to do, make”; query facio and retrieve inflected forms such as fecisset).[20] We also need at this stage translation services (e.g., a service that determines whether a given instance of the Latin word oratio more likely corresponds to “oration,” “prayer” or some other usage). At this point, knowledge based services augment general text mining (e.g., being able to cluster usages of the dictionary entry, facio, as a whole — or of facio as it is used in the subjunctive etc. — rather than treating each form of facio as a separate entity.)[21]
- Access to linguistically labeled, machine actionable knowledge: This overlaps with the analysis of visual structures but implies a greater emphasis on the analysis of natural language, e.g., “Y, son of Z,” “perf. feci” → the perfect stem is fec-., “b. July 2, 1887” → the subject of this encyclopedia was born in 1887 and any references to people by the same name that predate 1887 cannot describe this person,” etc. This stage corresponds to encoding information for particular ontologies, i.e., prescriptive structures separate from the text.[22] At this point, we should be able to pose queries such as “encyclopedia entries for Thucydides who is son_of Olorus or has_occupation historian, etc.”, “dictionary word senses is_cited_in Homer or has_voice passive;” “Book 1, lines 11-21 from all translations_of Homer_Iliad that have_language German.”
Fourth-Generation Collections
- Fourth-generation collections contain images of all source writings, whether these are on paper, stone or any other medium: Like third generation collections, the Cybereditions project sets out to incorporate page images of all print originals. Our goal is to help classical scholars shift the center of gravity for textual scholarship to a networked, digital environment. Scholars should not have to consult paper originals of scanned print editions to see what was on the original page.
- Fourth-generation collections manage legacy structures derived from physical books and pages but focus primarily upon logical structures that exist within and across pages and books: Even when fourth-generation collections depend upon page images, they exploit legacy book-page citations but they are fundamentally oriented towards the underlying logical structures within the documents. A great deal of emphasis is placed upon page layout analysis so that we can isolate not only tables of contents, bibliographic references and indices but dictionary and encyclopedia entries and critical scholarly document types such as commentary notes and textual apparatus. Cross language information retrieval hunts for translations of primary sources. Alignment services align OCR generated text to XML editions of the same works with established structural metadata. Quotation identification services spot commentaries by recognizing sequences of quotations from the same text at the start of paragraphs.
- Fourth-generation collections integrate XML transcriptions of original print data as these become available: All digital editions are, at the least, re-born digital: The best work published so far cannot convert the elliptical and abbreviated conventions by which scholars represent textual data in print into machine actionable data — we cannot even reliably link the textual notes to the chunks of text which they cover, much less convert these notes into machine actionable formats so that we could automatically compare the readings from one MS against those of another. Fourth generation collections naturally integrate page images with XML representations of varying sophistication. XML representations may, like first generation collections, capture basic page layout and they may have advanced structural and basic semantic markup (e.g., careful tagging for each speaker in a play). They may encode no textual notes, textual notes as simple footnotes (free text associated with a point in the reconstructed text) or as fully machine actionable variants (e.g., variants associated with spans of source text, such that we can, among other things, compare the text in various editions or witnesses).
- Fourth-generation collections contain machine actionable reference materials: Our digital collections should be tightly and automatically embedded in a growing web of machine actionable reference materials. If a new prosopography or lexicon appears, links should appear between its articles and references to the people or words in the primary sources. Commentaries should align themselves automatically to multiple editions of their subject work. To the extent possible, these links should bear human readable and machine actionable information: humans should be able to see from a link what the destination is about (e.g., “Thucydides the Historian” rather than “Thucydides-3,” ἀρχή-“empire” rather than “ἀρχή-sense2”). Equally important, these links should point to machine actionable information: a named entity system should be able to mine the entries in the biographical encyclopedia to distinguish Thucydides the Historian, Thucydides the mid-fifth century Athenian politician and various other people by that name; a word sense disambiguation system should be able to use the lexicon entries to find untagged instances where ἀρχή corresponds to “empire” or “beginning.” Editions should be self-collating — when a new edition of a text comes on-line, we should see immediately how it differs from its predecessors.
- Fourth generation collections learn from themselves: Even the simplest digital collection depends upon automated processes to generate text from page images or indices from text. Clustering and other text mining techniques discover meaning in unstructured textual data. Fourth-generation collections, however, can also learn from the machine actionable reference materials that they contain so that they apply increasingly more sophisticated analytical and visualization services to their content. In effect, they use a small body of structured data — training sets, machine actionable dictionaries, linguistic databases, encyclopedias and gazetteers with heuristics for classification to find structure within the much larger body of content for which only OCR-generated text and catalogue level metadata is available. In a fourth generation collection, structured documents are programs that services compile into machine actionable code: Aeneid, book 2, line 48 in a dozen different editions already on-line as image books with OCR generated text.
- Fourth generation collections learn from their users: Even third generation systems depend upon the ability of OCR software to classify markings into distinct letters and words. Fourth generation systems include an increasing number of classification systems such as named entity analysis, word sense disambiguation, syntactic analysis, morphological analysis, citation and quotation identification. Where there are simple decidable answers (e.g., to which Alexandria does a particular text refer?) we want users to be able to submit corrections. Where the answers are less well-defined (e.g., expert annotators do not agree on word sense assignment and some passages are simply open to multiple interpretation), we need to be able to manage multiple annotations. Human annotators need to be able to own their contributions and readers should be able to form conclusions about their confidence in individual contributors. Automated systems need to be able to make intelligent use of human annotation, determining how much weight to apply to various contributions, especially where these conflict. We therefore need a multi-layer system that can track contributions, by both humans and automated systems, through different versions of the same texts.
- Fourth generation collections adapt themselves to their readers, both according to specific recommendations (customization) and by making inferences from observed user behavior (personalization): Fourth-generation collections can process knowledge profiles that model the backgrounds of particular users: e.g., one user may be an expert in early Modern Italian, who has read extensively in Machiavelli, but only have a few semesters of classical Greek with which to read Thucydides and Plato. The fourth-generation collection can determine with tolerable accuracy what words in a new Italian or Greek text will be new and/or of interest, given the differing backgrounds but consistent research interests of the professor. At the same time, the system can infer from the reader’s behavior what other resources may be of interest.
- Fourth-generation collections enable deep computation, with as many services applied to their content as possible: No monolithic system can provide the best version of every advanced service upon which scholarship depends. Google, for example, has a growing number of publications about ancient Greece but currently produces only limited searchable text from classical Greek. Different groups should be able to apply various systems for morphological and syntactic analysis, named entity identification, and various text mining and visualization techniques with minimal, if any, restrictions. These groups should include both commercial service providers as well as individual scholars and scholarly teams.
The Classical Apographeme
- c. 500 “book-length” authors/collections. Hundreds and thousands of ancient Greek and Latin authors survive as names or with a small number of fragments preserved in quotations of later authors or on papyrus. F. W. Hall’s Handbook to Classical Texts lists 133 entries in its survey of the “chief classical writers” — including portmanteau works with many authors (e.g., the Greek Anthology) and authors with very large corpora (e.g., Aristotle and Cicero).[24] The Loeb Classical Library does not contain comprehensive editions for massive authors such as Galen or the early Church Fathers but its 500 volumes contain Greek and Latin texts as well as English translations for most surviving authors and works. If we assume that Galen and early church fathers would double the size of the Loeb, then we would have c. 500 volumes worth of Greek and Latin source text. Measured by word count, the corpora of classical Greek and Latin are closer to 100 and 20 million words respectively.[25]
- c. 1,000 manuscripts (MS) and an undetermined number of papyri, many very small fragments of literary works. Based on a survey of summary data from Richard and Olivier's Repertoire des bibliothèques et des catalogues de manuscrits grecs (1995), we possess more than 30,000 medieval manuscripts that contain at least parts of Greek classical texts (there are nearly 1,200 manuscripts for Aristotle alone). Since the number of extant Latin manuscripts is conventionally assumed to be 5 to 10 times that of Greek manuscripts, there might be as many as 150,000 to 300,000 manuscripts for Classical Latin. Nevertheless, a small subset of these provide most of the textual information relevant to the authors and editions of the most commonly studied authors. Hall’s early twentieth-century Handbook to Classical Texts summarized the major MS sources for major classical authors and contains c. 650 readily identifiable MS sigla (e.g., patterns of the form “A = Parisinus 7794”) — while editors have since added additional manuscripts of importance for most authors, Hall provides a reasonable estimate for the number of the manuscripts on which our editions primarily depend. Some authors do not have a few very authoritative MSS and editors must examine large numbers of MSS of roughly equal authority, and these will inflate the total. Assuming that this list underestimates the whole by 50-100%, we are still left with the evidence that a database of 1,000 MSS would represent the majority of textual knowledge preserved for us by MS transmission.
- c. 5,000 major editions over the five centuries extending from the editiones principes of the early modern period to the start of the twenty-first century. Assuming at the high end that each author has c. 10 volumes worth of major editions. Multi-work canonical authors will have many editions of individual and selected works. At the very high end, the New Variorum Shakespeare series chooses c. 50 editions of each play as worth collation and this may represent an upper bound for canonical texts outside the Bible.
- c. 5,000 translations in European languages such as English, French, German and Italian. These are important because we can use parallel text analysis to infer translation equivalents and word senses and then use advanced language services (e.g., syntactic analysis, named entity analysis) on the translations and then project this backwards onto the original. Such a technique can, for example, add 15% to our current ability to analyze Latin syntax (e.g., from 54% to 70%).
- c. 5,000 modern commentaries, author lexica etc. These are useful for human readers and may lend machine actionable data as well.
- c. 1,000 general reference works such as lexica, grammars, encyclopedias, indices and other entry/labeled paragraph reference works with high concentrations of citations and, in some cases, elaborate knowledge bearing hierarchical structures.
- c. 1000 specialized studies of Greek and Latin language in a sufficiently structured format for high precision information extraction.
Three Technical Challenges
-
Leverage the fact that many historical texts quote documents for which
excellent transcriptions exist in machine-readable form
Thus, the tenth century Venetus A manuscript (Figure 1 and Figure 2) and Jensen’s 1475 incunabulum (Figure 3 and Figure 4) contain texts of Homer and Augustine. We need systems that can use their knowledge that a given document represents texts for which transcriptions exist to decode the writing system of the document, to separate text from headings, notes, and others annotations, to recognize and expand idiosyncratic abbreviations of words within the text, to distinguish variants from errors, and to provide alignments between the transcribed text and their probable equivalents on the written page. Even if we only succeed in general alignments between a canonical text and sources such as early modern printed books and manuscripts, the results will be significant.[26] If we can improve our ability to collate manuscripts or extract useful text from otherwise intractable sources, the results will be powerful.This task requires very different OCR technology from that currently in use. In this case, we assume that our texts contain many passages for which we possess good transcriptions. The problem becomes (1) finding those quotations, (2) learning what written symbols correspond to various components of transcription, and (3) comparing multiple versions of the same passage to distinguish variants and errors. The OCR system uses a library of known texts to learn new fonts, idiosyncratic abbreviations and even handwriting.There are two measures for this category of OCR. First, there is the overall character accuracy of transcriptional output from documents that the OCR software produces by training itself with recognized quotations. Second, the ability to locate quotations of existing texts is an important scholarly task in and of itself.[27] Two of the prime tasks in the German eAqua Classics Text Mining Project focus on identifying undiscovered quotations of Plato and of Greek Fragmentary Historians.[28] The apparatus criticus for the Ahlberg Sallust (Figure 9 and Figure 10), for example, includes not only textual variants but testimonia — places where later authors have quoted Sallust. Such manually constructed lists of testimonia provide us with instruments with which to measure precision and recall for automated methods.
-
Use propositional data already available to decode the formats in which
unrecognized knowledge has been stored.
Printed reference works contain an immense body of information that can be converted into machine actionable knowledge. The Perseus Digital Library, to take one example, has tagged hundreds of thousands of propositional data within reference works originally published on paper. Thus, the Liddell-Scott-Jones Greek-English (Figure 13 and Figure 14) and Lewis and Short Latin-English lexica, for example, contain tagged citations to 422,000 Greek and 303,000 Latin authors (i.e., citations tagged with author numbers from the TLG and PHI canons of Greek and Latin authors). Since the structure of the dictionary articles has also been tagged, many of these citations represent propositional statements of the form SENSE-M of DICTIONARY-WORD-N appears in CITATION-P of AUTHOR-Q.The works of many Greek and Roman authors survive only insofar as other authors have quoted or described them. These fragmentary texts are published as lists of excerpts (Figure 12). Thus, fragment 116 of the historian Ephorus in Mueller’s edition contains an excerpt from chapter 12 of Plutarch’s Life of Cimon. Each of which represents the propositional statement “EXCERPT-A frm CITATION-C of AUTHOR-D refers to (fragmentary) AUTHOR-X.” Note that not all citations refer to the author: thus, fragment 113 of Ephorus includes a cross-reference for background information on a historical event in Herodotus, who wrote before Ephorus.Grammars also contain well-structured information: citations within a section on contrary to fact conditionals, for example, (Figure 15 and Figure 16 through Figure 18) can be converted into propositional form: e.g., GRAMMATICAL-STRUCTURE CONTRARY-TO-FACT occurs at Xenophon’s Cyropaedia, book 1, chapter 2, section 16. Fine-grained analysis of the print content can also extract quotations and their English translations that appear throughout reference grammars and lexica. Smyth’s Greek Grammar, the German Kühner-Gerth reference Greek Grammar, and the Allen and Greenough Latin Grammar contain 5,300, 21,000 citations and 2,000 tagged citations within labeled sectionsCitations in indices of proper names and in encyclopedias about people and places provide similar propositional data to disambiguate references to ambiguous names: thus, the print index to Rawlinson’s Herodotus (Figure 20) distinguishes passages where Herodotus cites Alexander, a king of Macedon, from Alexander, the son of Priam who appears in the Trojan War. Encyclopedias (Figure 22) contain citations from many different sources and many different people and places with the same name. By converting the citations to links and then extracting the contexts in which different Alexanders appear, machine learning algorithms can be used to find patterns with which to distinguish one Alexander from another elsewhere. The Smith’s biographical and geographical dictionaries contain 37,000 tagged citations for 20,000 entries on people and 26,000 tagged citations for 10,000 entries on places. The Perseus Encyclopedia, integrating entries from originally separate print indices contains 69,000 citations for 13,000 entries.A great deal of information remains to be mined from the print record and we need to be able to leverage the information already extracted to extract even more from the much larger body of reference materials available only as page images.Extraction contains at least two dimensions. In each case, we need more scalable methods.
- Parsing the structure of individual documents: Even if we can recognize that “Th. 1.33” represents a citation to a text, we need to determine whether this cites book 1, chapter 33 of Thucydides’ Peloponnesian War or Idyll 1, line 33 of Theocritus. The indices shown at Figure 20, Figure 21, Figure 15, etc. illustrate some of the varying formats with which different works encode similar information
- Aligning information from different documents: Author indices distinguish different people and places with the same name in the same document, but aligning information from multiple author indices is not easy. Is Alexander the son of Amyntas in Herodotus the same person as Alexander the father of Perdiccas in Thucydides?
-
Use existing translations of source texts to generate multi-lingual
services such as cross language information retrieval, word sense
disambiguation and other searching/translation services.
There are already English translations aligned by canonical citation to more than 5,000,000 words of Greek and Latin available in the Perseus Digital Library. These provide enough parallel text to support basic multi-lingual services such as contextualized word glossing (e.g., recognizing in a given context whether oratio is more likely to correspond to “prayer,” “oration” or some other word sense), cross language information retrieval (e.g., being able to generate “prayer” and “oration” as possible English equivalents of Latin oratio), and semantic searching (e.g., find all Latin and Greek words that probably correspond to the English word “prayer” in particular passages).The larger our collections of parallel text and translation, the more powerful the services can become. We need methods to locate more translations of Greek and Latin and then to align these with their sources. In some cases, library metadata will allow us to identify translations of particular Greek and Latin works. In other cases, however, we will need to depend upon cross-language information retrieval to find translations where no machine actionable cataloguing exists (e.g., anthologies, quotations of excerpts or smaller works).Once we have identified a translation, we need automated methods to align translation and text. Figure 11 shows a best case scenario: a book where the modern translation and classical source text are printed side by side. In this case, the modern translation shares the chapter number of the Latin source text (both have “LXIV” to indicate that they include chapter 64), but the English translation does not include the finer grained section numbers in the Latin text. We need automated methods to align the many translations now appearing in large image book collections.
Conclusion
Appendix: Sample Page Images
Primary Sources
The 10th Century Venetus A MS of Homer
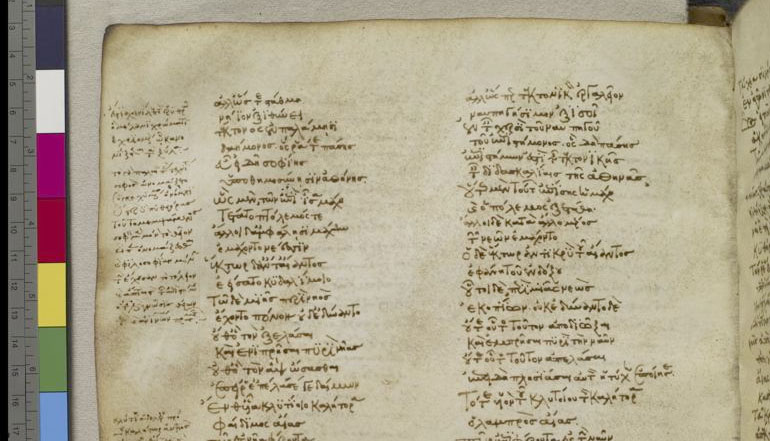
The 1475 Jensen printing of Augustine’s De Civitate Dei
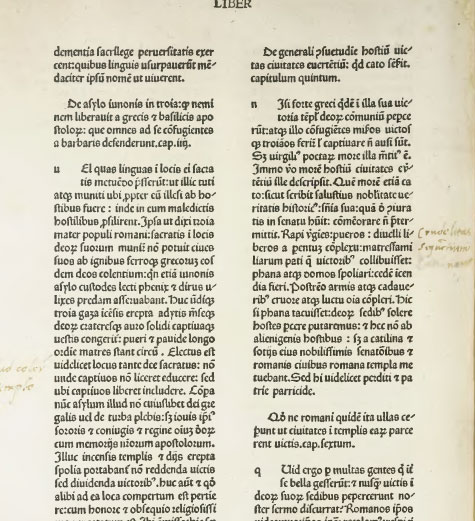
Tyrrell’s Edition of Cicero’s Letters
Ahlberg’s 1919 Edition of Sallust
Translations
Editions of Fragmentary Authors and Works
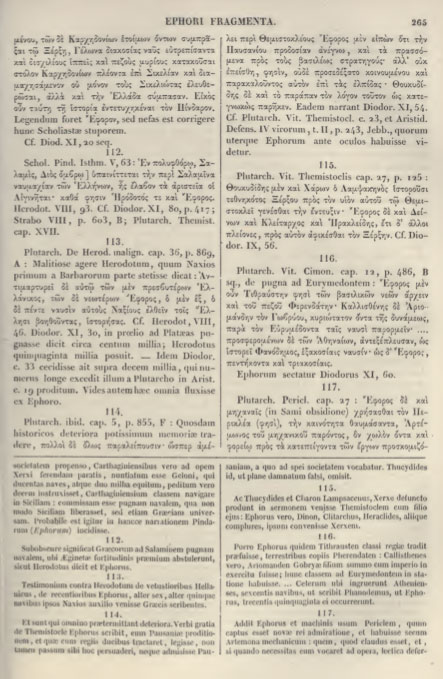
Reference works
Lexica
Lidell Scott Greek-English Lexicon
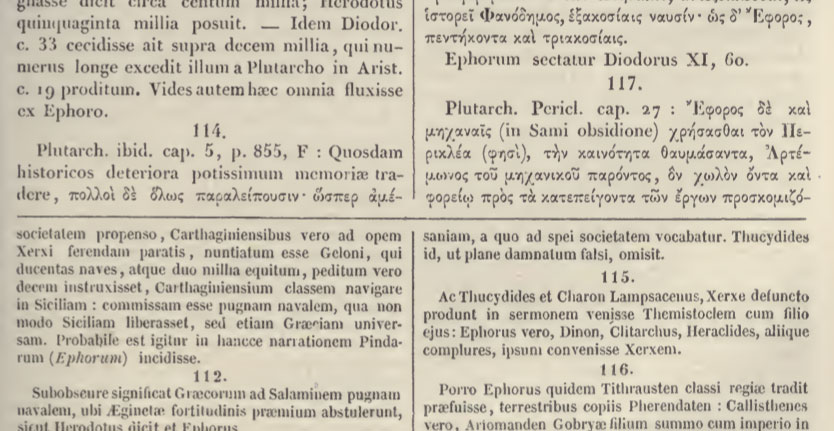
Grammars
Goodwin and Gulick’s Greek Grammar
Rutherford’s First Greek Syntax
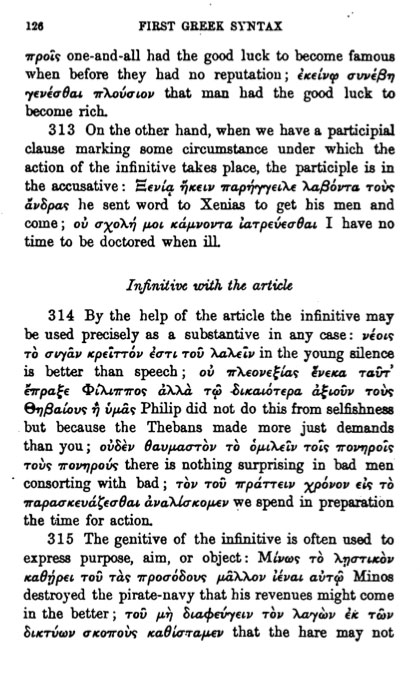
Information about People, Places, Organizations and other Named Entities
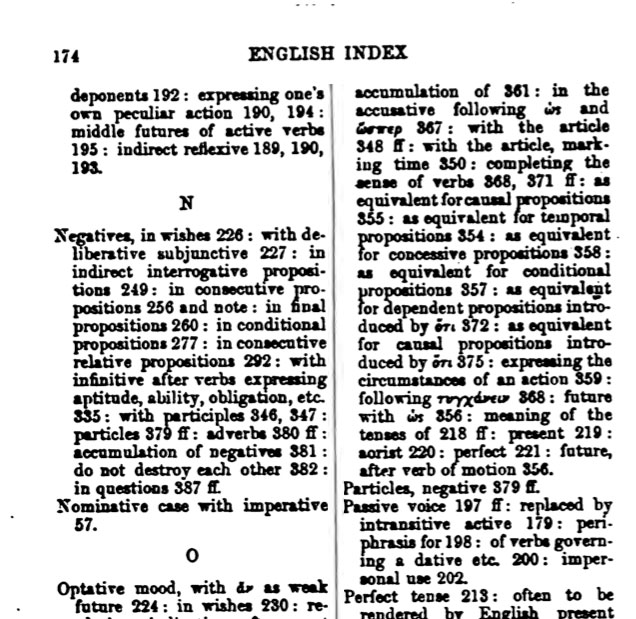
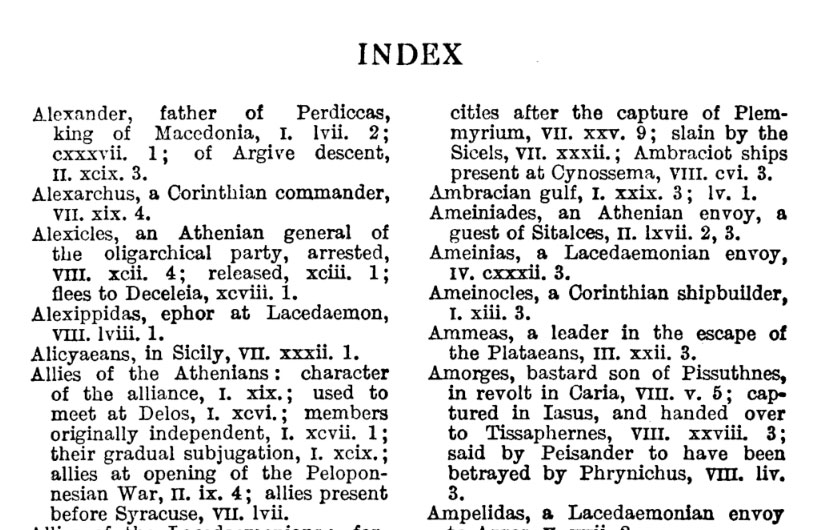